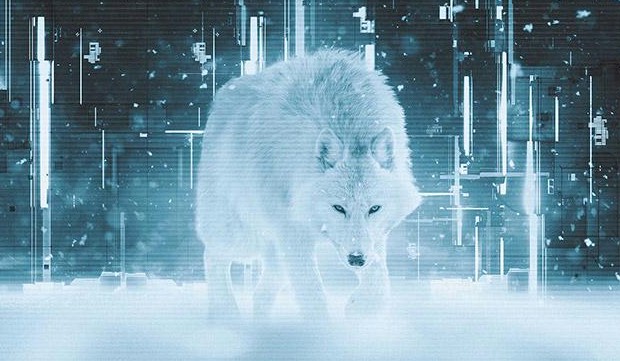
Success in any endeavor is not just about having the right idea, but having that idea at the right time and then executing well against that plan.
It is safe to say that in the traditional HPC simulation and modeling arena, the combination of the “Shasta” Cray EX supercomputer line, now owned by Hewlett Packard Enterprise, and the Epyc CPUs and Instinct GPU accelerators from AMD, which has dropped the “Radeon” brand from their name, is on a serious roll. Every week it seems there is a new deal or two for a big supercomputer that is based on the Shasta design and its Slingshot variant of Ethernet paired up with future AMD CPUs and GPUs. It is hard to keep up, and the second one that was announced this week is an important one given its size and scope. And rather than just drive through the details of the first of the three pre-exascale systems commissioned by the European Union under its EuroHPC effort, which is variously called Snow or LUMI and which will be installed in Finland, we want to use this announcement to ask a larger question:
What is going on here?
And so, before we dive into the details of the Snow system – LUMI is short for Large Unified Modern Infrastructure and we think is a backronym given that Lumi is a girl’s name in Finnish that translates into English as Snow – we want to make some observations about what might be going on in the HPC market that explains the wild success that Cray and AMD are having together despite intense (and increasing) competition from Nvidia for compute engines and the emergence of a CPU-GPU alternative from Intel in the coming years.
The largest HPC centers are often a leading indicator for technologies that will see wider adoption in a broader IT market – but it is important to not get too carried away. AMD and Cray certainly had a lot of success together in HPC with the Opteron line of processors and the proprietary “SeaStar” and “Gemini” interconnects that Cray created to lash them together, and this presaged the broader adoption of Opteron processors in the enterprise. Because of missteps on the part of AMD and increasing competition between AMD and Intel, where Intel delivered a better processor after mimicking the Opteron design with its “Nehalem” Xeon E5500s in early 2009, AMD was basically shut out of HPC and then the enterprise for the better part of a decade. What goes up often comes down. But in this case, the rise of AMD along with Cray in HPC might be a different story: What goes up sometimes stays up and becomes the new normal.
Let’s start with a characterization of the high performance computing market in a very general and broad sense, including both traditional HPC simulation and modeling workloads as well as AI and data analytics workloads that are increasingly running within or beside HPC applications. We think there might be three (or more) high performance computing camps emerging.
In the past, there were what the HPC market watchers at Hyperion Research (formerly of IDC) called capability-class supercomputers, which meant those that pushed the bleeding edge of architecture and performance to generally run a relative handful of workloads at extreme scale. And this contrasted with what they called capacity-class supercomputers, which were designed to run a slew of relatively small jobs. Most of the systems we call supercomputers, including those in most government, academic, and enterprise institutions, are of the latter kind, and it is no surprise that many of these customers are able to shift their work to the public cloud sometimes. It is also no surprise why the former, who often are simulating nuclear weapons and who need extreme scale – always, always, always more than they can ever get – cannot move to the public cloud.
We would observe that in a very real way, these differences in the HPC market – capability versus capacity – were replicated, in a slightly different form, in the Internet arena by what we call hyperscalers and cloud builders.
The hyperscalers run free and often advertising supported applications at absolutely ginormous scale – unlike anything any HPC center in the world has ever been able to get funding for, millions of nodes versus thousands – but they typically only have a handful of core applications that soak it up. Just like capability-class HPC systems generally installed at the national labs around the world. The hyperscaler clusters run search engines, advertising systems, data analytics, or machine learning workloads that span tens of thousands of nodes at a time, and in a very real sense they have many of the same problems as the capability-class HPC crowd. But because hyperscale infrastructure is designed from the ground up, often by people who hail from Stanford University, the University of California at Berkeley, and a few other notable academic institutions with deep expertise in processors, interconnects, and distributed systems such as file systems, databases, and compute frameworks, they have generally created their own analogs to whatever the HPC community had already long since created.
The public cloud builders, on the other hand, are generally supporting Windows Server and Linux workloads running on X86 servers, and in a very real sense, they build what are more like capacity-class supercomputers in that their clusters are chopped up into virtual machines or containers to run specific customer environments and the game is to cram as many of these customers onto a single machine. Just like a capacity-based supercomputer, which slices its capacity up using the Message Passing Interface (MPI) protocol and tries to get as many simulation and modeling jobs crammed onto the system at the same time to get peak, sustained utilization at all times.
As we sit here and watch the next generation of pre-exascale and exascale systems being announced, we might be witnessing another split in the high performance computing market. And again, we are using that term, HPC, very generally and not specifically restricting it to traditional HPC simulation and modeling but also including various AI and data analytics workloads that are more and more common in enterprises and HPC centers and which have been largely driven by the needs of the hyperscalers to improve their consumer-facing services.
To be specific, we might be seeing a bifurcation – or even trifurcation – of high performance computing organizations:
- First, there are those that focus on traditional HPC and need that to run at scale with the lowest possible dollars per flops, no matter what the bit-ness of the applications and data is, and who have some AI processing needs but this is a secondary consideration.
- And then there are organizations who need the absolute best AI performance and all they are really doing is AI. And those that need to do AI right now are by and large almost universally choosing Nvidia GPUs and AMD CPUs to do it – and the latter is more about the “Rome” Epyc chips supporting PCI-Express 4.0 than a choice of AMD Epycs over Intel Xeon SPs. When Xeon SPs are in the field later this year and next with credible core counts, performance, and PCI-Express 4.0 interconnects to accelerators, we shall see.
- And finally there are organizations who are doing AI but also need to do traditional HPC on their supercomputers, but the AI performance is primary and the HPC performance is secondary. So here, you might see the selection of compute engines that are a little light on the 64-bit floating point precision, but heavy on the other math.
Within each of these segments, there are capability and capacity distinctions to be made, perhaps.
What seems clear at this point, in watching the resurrection of the AMD-Cray partnership and the re-emergence of AMD as a compute engine supplier to traditional HPC shops, is that raw performance and low price still matter here. Bigtime. There is nothing about the Cray Shasta design that requires customers to pick any particular CPU, GPU, or interconnect. But of the recent deals, almost all of the systems are using the Slingshot interconnect and AMD CPUs and usually GPUs, too. Slingshot looks and feels like Ethernet because it is Ethernet, albeit with HPC extensions as well as congestion control and adaptive routing, so that makes it an easy choice for customers who want to just manage one type of network in the organization rather than have to have InfiniBand or Omni-Path at the heart of the supercomputer and Ethernet everywhere else. (This was always Cray’s plan, and now HPE is benefitting from it mightily.) And while the future Epyc CPUs and Instinct GPUs used in many of these pre-exascale systems as well as the two exascale systems in the United States – that would be the 1.5 exaflops “Frontier” system at Oak Ridge National Laboratory coming in 2021 and the 2.1 exaflops “El Capitan” system at Lawrence Livermore National Laboratory coming in 2022 – are not specified in any detail, we presume that AMD won the compute engine part of the deal because it was offering not only more performance than Nvidia or Intel, but also at a lower price and therefore a considerably better value for the dollar.
If not, the US Department of Energy would have stuck with IBM Power10 CPUs and a future Nvidia GPU and a future Mellanox (now Nvidia) InfiniBand rather than risk a new CPU, a new GPU, and a new interconnect all at the same time. El Capitan, for instance, was specified to be a 1.5 exaflops machine, but for the same $600 million, HPE/Cray and AMD are delivering north of 2 exaflops, which we are guessing means 2.1 exaflops peak.
The Lumi system being installed at the CSC datacenter in Kajaani, Finland under the auspices of the EuroHPC effort is benefitting from all of the work that Cray and AMD did, and then HPE provided the financial might to deliver, to win those two exascale deals in the United States. And you can bet that if EuroHPC had a choice, there would be indigenous Arm and RISC-V engines in this machine, but Brexit messed up the European angle for opting for Arm (Great Britain will soon no longer part of the European Union). So given that a pre-exascale machine needed to be built next year, and it needed to be a big one, the least risky thing to do is to do what the United States is doing and still get an X86-based, capability-class supercomputer that can be shared like a capacity-class machine across many countries. The consortium behind the Lumi installation at CSC includes funding from Belgium, the Czech Republic, Denmark, Estonia, Finland, Iceland, Norway, Poland, Sweden, and Switzerland, and presumably, compute cycles are allocated in proportion to investments or GDP or some other metric.
Lumi will have a peak theoretical performance of 552 petaflops, and it will be partitioned in this fashion:
The precise configurations of the various partitions of the Lumi system were not divulged. But when Lumi was first talked about conceptually in May 2019, the idea was to have a GPU accelerated node in the Lumi-G GPU partition have one or two processors and four GPUs. We strongly suspect that this node configuration will be very similar to that of Frontier and El Capitan, which have one Epyc CPU paired with four Instinct GPUs. The promise was to have over 200 petaflops in this partition, and the final deal is to have 550 petaflops, so whatever happened, there is a lot more oomph in the GPUs. Using the Slingshot interconnect to link the GPU nodes to each other, the network has a global bandwidth of 160 TB/sec; Slingshot, of course, is running at 200 Gb/sec per port. We know that Lumi will use the “next-generation of AMD Instinct GPU processors,” of which the chip maker has said basically nothing.
The Lumi-C partition, also a core compute part of the machine but probably not with much floating point oomph compared to the GPUs, was to be an X86 node with medium, large, and extra large memory configurations on the nodes. That meant only Intel and AMD were in the running, and we now know AMD won and that this CPU partition will have around 200,000 cores. We strongly suspect that it will be comprised of two-socket Epyc CPU nodes to het a larger memory footprint per node without having to use very expensive 128 GB or 256 GB DDR4 memory sticks. What we do know is that this compute partition will be based on 64-core processors using “next generation AMD Epyc processors,” which means the forthcoming “Milan” chips due any day now. Probably around the SC20 supercomputing conference, if we had to guess. Assuming reasonable clock speeds and that this also uses 64 core parts, this partition could easily make up the other 2 petaflops – if those performance numbers are not just careless rounded in parts of the announcement by EuroHPC. If you had 64-core Milan parts running at top speed, you might be in the 8 petaflops zone for this CPU-only partition of Lumi.
The Lumi-D data analytics partition has 64 Instinct graphics cards and 32 TB of memory across that visualization, pre-processing, and post-processing partition. That memory is a mix of CPU and GPU memory to be that large. (If AMD could get 48 GB or 64 GB of HBM2 memory on a GPU, that would be only 3 TB or 4 TB of GPU memory, so the rest has to be coming from somewhere.) We wonder if this is actually a Superdome Flex system (based on the acquired SGI “UltraViolet” NUMAlink interconnect, with GPUs in the box. This would make sense, and in which case it is a NUMA machine with 32 TB of capacity and the GPU memory is ancillary and not counted.
As for storage, there is an all-flash cluster based on Cray ClsuterStor E1000 arrays that will have 2 TB/sec of I/O bandwidth and that will weigh in at 7 PM of capacity. There is also an 80 PB Lustre file system that will be entirely based on disk drives, and a 30 PB object store based on Ceph software and presumably also based on disk drives. That’s 117 PB of storage in total.
Lumi will be installed in the second quarter of next year, and should be among the highest performance systems in the world. A lot depends on when systems in the United States and China are fired up. Japan already has its big machine, the top-ranked “Fugaku” system that weighs in at 513 petaflops peak at double precision floating point.
The European Union has budgeted more than €960 million ($1.13 billion) for EuroHPC system procurements for 2019 through 2020 inclusive, and the Lumi machine represents more than €200 million (call it at least $237 million) for Lumi alone. Half of the funding is coming from the European Union and half from the participating EuroHPC countries – akin to federal and state funding in the United States and Australia, more or less. Here is how Lumi stacks up, very roughly, against the new machine at Pawsey Supercomputing Center in Australia, which we reported on earlier this week, and Frontier and El Capitan in the United States:
The Pawsey machine, also based on AMD CPUs and GPUs and the HPE Shasta design with the Slingshot interconnect, has more CPU compute – the word we hear is that only half the nodes have GPUs on this box, something we didn’t know earlier this week – and therefore the cost per unit of compute is higher and the performance per dollar is much lower. But you have to build the machine for the applications you expect, and this says as much about the applications these centers want to have as anything else.
Here is what this also suggests. For a lot of HPC centers, switching to Ethernet as long as it has HPC features is desirable, and they are also looking for the cheapest possible CPU and GPU compute engines.
The question now is this: Can AMD and the HPC labs together help create an AI platform that can rival that of Nvidia or at least emulate it well? Because if it can do that – and this is clearly AMD’s plan with the labs helping out with development – then maybe, just maybe, AMD can start eating into Nvidia’s AI share before Intel enters the fray with Xeon SP processors, Xe GPU accelerators, and its oneAPI programming stack.
“so that makes it an easy choice for customers who want to just manage one type of network in the organization rather than have to have InfiniBand or Omni-Path at the heart of the supercomputer and Ethernet everywhere else”
..so true and so welcome