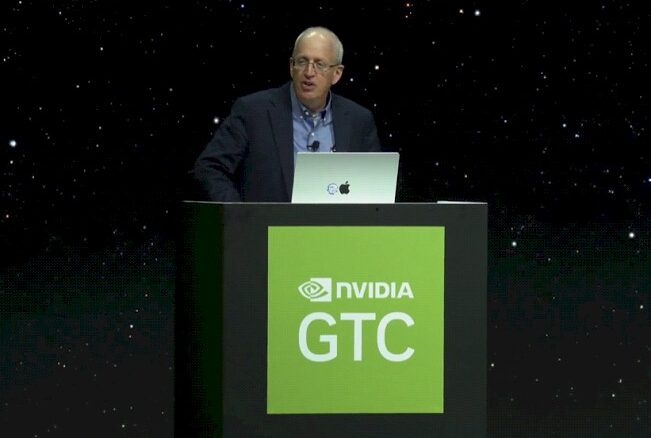
It is safe to say in 2025 that the best job in the world is the chief executive officer of Nvidia, and that the company’s co-founder, Jensen Huang, has steered the company to great heights as much as fellow co-founders Thomas Watson ever did with International Business Machines, Larry Ellison ever did with Oracle, and Steve Jobs ever did with Apple Computer.
But the second best job at Nvidia for sure is the one that Bill Dally holds. Or rather, the two jobs Dally does at the same time. As Nvidia’s chief scientist, a role Dally assumed in 2009 after being chairman of the computer science department at Stanford University for a dozen years and an illustrious career in chips and interconnects and systems at MIT and CalTech for decades before that, Dally says he pokes his nose in everything that is going on around the company and tries to make all of that technology “as good as it can be.” In his presentation at the latest GPU Technical Conference in San Jose, Dally added that he has another job running Nvidia Research, which means he gets to “work with a lot of really smart people on a lot of hard intellectual problems.”
Dally’s presentations are always interesting, and the one at the most recent GTC was no exception. As usual, Dally picked a few neat technologies in hardware and software and drilled down into them and either showed how they have given Nvidia a current edge or might in the future when it comes to selling GPU accelerated systems.
That got us to thinking about Nvidia’s research and development spending, which is pretty hefty but which now looks miniscule thanks to the explosion in Nvidia’s revenues and profits in the past year and a half. But make no mistake: Nvidia has invested heavily to create the future that it is now benefitting from more than any other vendor on planet Earth.
Back in November 2008, when Nvidia first articulated its GPU compute aspirations in the HPC simulation and modeling arena at the Supercomputing 2008 conference in Austin – this was nearly a year before the first GPU Technology Conference was held at the Fairmont Hotel with about 1,500 attendees –IBM’s “Roadrunner” hybrid supercomputer based on AMD Opteron CPUs and IBM PowerXCell math accelerators had just broken through the petaflops barrier on the High Performance LINPACK benchmark the previous May. Just to show you how far away we were from the AI revolution and our current realities, Pacific Northwest National Laboratory hosted a panel discussion called “Will Electric Utilities Give Away Supercomputers With The Purchase of A Power Contract?” ( I attended this session and still have photos of the presentation and Dan Reed, who had just joined Microsoft from the National Center for Supercomputing Applications at the University of Illinois a year early, holding some now obscure computing device in his hand.)
The reality has turned out far different: Will You Pay More For A Supercomputer And A Power Contract Than You Ever Imagined You Could Get Budget For? Perhaps we will propose this as a panel discussion to the SC25 folks. And that reality is utterly dominated by the performance, power consumption, and cost of Nvidia datacenter GPUs.
And, we contend, part of the reason that future turned out different is that the Nvidia GPU became the workhorse parallel computing engine for HPC, analytics, and AI workloads, even though CPUs with vector and sometimes tensor engines still do a lot of parallel work, too.
None of that would have happened if Nvidia didn’t see the value in the Brook Stream processing programming language, which offloaded math calculations to the floating point shader units on ATI (now AMD) and Nvidia GPUs and hire its creator, Ian Buck, to create what is now called CUDA. The needs of GPU compute in the datacenter have forced the GPU design to evolve and its programming stack along with it. And when the world had created enough data that AI algorithms from the dawn of time (the early 1980s) would actually work, the GPU was the best compute engine to do that work and became the platform for the AI revolution – and specifically, we mean classical machine learning. GenAI and its foundation models are just the second wave in what will very likely be many waves of AI innovation. And it is Nvidia Research, under the watchful eye of Dally, that is making sure that GPU chips evolve and that the workloads that might do well with massive amounts of parallel compute can be married to future GPUs and keep the technology flywheel – and therefore the money flywheel – spinning.
Put It On My Bill
This has taken investment. We only started tracking Nvidia’s financials back in April 2010, which is the first quarter of its fiscal 2011, because its datacenter business was not even yet material. Nvidia did not even report Datacenter division revenues until the first quarter of fiscal 2015, and it was a mere $57 million at the time against total sales of $1.1 billion at the time and net income of $137 million. Not for nothing – and we really mean that – Nvidia spent $337 million in that quarter – 30.6 percent of revenue – on research and development, and had been beefing up its R&D spending to new heights for the prior two years as a percent of revenue, peaking at 34.2 percent of revenues in Q1 F2014.
This was Nvidia laying the groundwork to capitalize on the first wave of the AI and to get into position for the second wave. And rest assured, it has prognosticated the third wave (which it calls physical AI), and it is no doubt preparing for whatever the fourth wave is.
Now, to the inexperienced eye, it may look like Nvidia is cutting way back on R&D spending and we should be worrying about its investment in the future. Not true. The fact is, Nvidia has been able to corner the market on GPU compute because of its two decades in creating the CUDA platform, a collection of over 900 libraries, frameworks, and models that underpin every accelerated HPC and AI application in the world. Another fact is that Nvidia can pay the absolute top dollar for the scare HBM memory that modern datacenter GPUs require to run AI training workloads, and increasingly AI inference because of the intense computational needs of chain of thought or reasoning models.
If HBM were not so scarce and expensive, AMD would be stacking DRAMs up to heaven against its MI250 and MI300 GPUs and would be selling a whole lot more GPUs than it currently does. But HBM is scare and AMD cannot pay as much as Nvidia can. Which is why we didn’t see AMD project $10 billion or $20 billion of GPU sales in 2025 after making more than $5 billion in 2024.
But, for a certain subset of users – the HPC crowd – the CUDA X stack, as that Nvidia software is called, is not as important as it has been for the AI crowd, who stands upon the shoulders of the HPC crowd no matter how much they protest otherwise. (NCCL is a gussied up MPI, for instance.) And this is why you see AMD pursuing traditional HPC centers with its GPUs, and getting traction there because HPC centers are, among other things, extremely price sensitive when it comes to compute. AI customers, who do their computing to make models that will hopefully make money, can line up any number of investors to make it happen. HPC centers rely on state and national governments.
If you look at the past decade and a half, Nvidia’s R&D spending has been somewhere between 20 percent and 25 percent of revenues, which is on the order of what Meta Platforms has done since it took over designing its own servers, storage, networking, and datacenters over that same time. Google tends to spend somewhere between 15 percent to 20 percent of revenue on R&D, as does Oracle. Microsoft is about 15 percent, give or take a smidgen, and Amazon is a few points lower. AMD used to spend between 15 percent and 20 percent, but now it is the same bracket as Nvidia. But, in the trailing twelve months, Nvidia had $130.5 billion in sales, a factor of 5.1X larger than the $25.8 billion that AMD brought in.
That said, even though Nvidia has kept growing its R&D budget during the GenAI boom, it is not spending 20 percent to 25 percent of revenues on R&D. In fact, it has been trending down as a share of sales since the summer of 2023, when the GenAI Boom sent Nvidia revenues and earning skyrocketing. It has averaged just under 10 percent of revenues in the trailing twelve months. But that still represents 48.9 percent growth comparing fiscal 2025 to fiscal 2024, and a total of $12.91 billion in R&D.
This log chart shows you better how steady the R&D spending increases have been at Nvidia:
We don’t know how much of that figure is R and how much is D, but we think that as Nvidia takes over more and more of the datacenter hardware and software stack, there is a steadily growing amount of R investments and an explosion in D costs. It is hard to say with any precision.
As Dally pits it, Nvidia Research is roughly dividing into two parts, what he called the supply side and the demand side.
The supply side involves research in everything from circuits all the way up to system architectures, with the explicit job of supplying “the technology that makes GPUs great,” as he put it. This supply-side research now includes GPU storage systems and security, which are integral to any commercial AI system.
The demand side is about doing research into all kinds of application areas so that the universe of accelerated computing keeps expanding, thereby driving up demand for Nvidia GPUs. There are two different AI groups, one in Toronto and the other in Tel Aviv, and another in Santa Clara that does applied deep learning research. The lab in Taiwan is where Generative AI work is done as well as multimodal learning and 3D vision. There are specialized AI labs that focus on robotics and autonomous vehicles, and other groups that focus on large language models or efficient AI algorithms. There are apparently three groups that focus on graphics and one that does quantum physics and chemistry.
Nvidia Research has just formed a quantum computing study group that is trying to assess the current state of that technology and see where and how and when Nvidia might play in the opportunity.
And everyone once in a while, there is what Dally calls a moonshot, where researchers from all over the Nvidia Research organization and across the product divisions to bring a new technology to life. The RT cores that are part of the graphics cards (and therefore some inference cards sold into the datacenter) and that are used to speed up the processing of ray tracing is one example of a moonshot project. That project started in 2013 and RT cores made it into the “Turing” GPUs in 2017.
Nvidia probably has somewhere around 500 researchers that are formally part of Nvidia Research, but has thousands of additional engineers from the product groups that also are part of certain projects. Nvidia has around 36,000 employees right now, and we estimate that 75 percent of them work on software, the traditional share of the Nvidia workforce over at least the past decade.
One of the most successful technology transfers out of Nvidia Research into the product divisions was NVLink and NVSwitch, which is something we have discussed before. But in his keynote at GTC 2025, Dally elaborated a little further:
“I actually got a contract from the Department of Energy in about 2012 or so, back when we were building supercomputers for Oak Ridge,” Dally explained, referring to the contract for the “Summit” supercomputer for which IBM was the prime contractor. “As part of those programs, there was research and development funding. So I applied for some to develop a GPU network. And I remember, at the time, the Department of Energy wanted to cost share the project. They wanted to have Nvidia pay 40 percent and the Department of Energy pay 60 percent. I went to Jensen and he said, “Absolutely not. Yeah, we don’t do networking. We’re a GPU company.” So I went back to the Department of Energy, and fortunately, 100 percent they funded the project to develop the first NVSwitch and the first NVLink. And from there, actually, those projects got grabbed out of our hands before we were done with the project, and they realized they needed to make several GPUs look like one big GPU. And Nvidia has been involved in networking ever since.”
But Dally said probably the most important technology transfer for Nvidia Research is machine learning.
“So I got Nvidia Research involved in machine learning in 2011 after having had breakfast with my Stanford colleague, Andrew Ng,” Dally recalled. “He was telling me at the time he was at Google Brain finding cats on the Internet using 16,000 CPUs. And I thought, gee, we could do this with GPUs and take a lot fewer. So I assigned Brian Catanzaro, who the time was a programming system researcher, to work with Andrew, and he ported that software to run on 48 GPUs, and actually ran faster on 48 GPUs than on 16,000 CPUs. And that software became cuDDN and launched us down the path where we are today with deep learning.”
There have been a lot of technology transfer successes over the years, and here is a small sample:
One of the technologies that Dally talked about at this GTC was ground-referenced signaling, which he talked to us way back at Hot Chips in the summer of 2019. GRS is a single-ended signaling technique and, to simplify it a whole lot, allows Nvidia to drive twice the bandwidth per pin through the wire traces on an organic substrate at twice the bandwidth per pin as other differential signaling techniques and at twice the bandwidth per millimeter of the edge of the chip. And, here we are six years later, and GRS signaling is being used to link Nvidia’s “Grace” CG100 CPUs to the impending “Blackwell” B300 GPUs in the DGX GB300 systems.
Back in 2013, when the GRS research was just starting, Nvidia could do 25 Gb/sec signaling at around a half picojoule per bit, but to make the signal more robust, it boosted it to around 1 picojoule per bit with the production GRS. A typical PCI-Express link, says Dally, needs on the order of 5 picojoules to 6 picojoules per bit for its signaling.
Dally just get tossing new ideas out that we can expect to end up in products. Here’s inverter based signaling on an interposer, where you might be, for instance, linking multiple GPU chiplets to each other on a single package:
And here is an Nvidia approach to an interconnect for 3D chip stacking:
And here is how this 3D stacking might compare to interposers in terms of bandwidth per pin and the femtojoules per bit consumed to push the signals:
It just goes on and on and on, as you can see from watching Dally’s Nvidia Research presentation at GTC 2025 and indeed the ones he has made over the years and various events.
Back in the day, when IBM was so rich it could indulge in just about any research it wanted to, it did so. And then, when the business went up on the rocks in the early 1990s and Big Blue was three hairs away from bankruptcy, one of the first things that Louis Gerstner, the only IBM chief executive officer brought in from the outside, did was to get IBM Research focused only on solving real problems aimed at issues affecting real customers.
Nvidia never had to refocus its researchers and engineers to do that. This is all that they have ever done. Hopefully, as Nvidia is now very rich in terms of revenue streams and net income pools, the company does not overindulge in research, fearing it will miss whatever next wave might be coming. All it need do is stick to its GPU knitting, and its roadmap shows that is the plan.
One more thing to consider: Nvidia doesn’t invent everything, but it does invent the things it believes it needs to differentiate from the competition or establish new markets. So, for instance, Nvidia buys PCI-Express switches and retimers from third parties. It buys DRAM, GDDR, HBM, and flash memory from multiple suppliers. It has preferences for specific use cases for technology brought in from the outside, and neutrality where it is warranted. It will buy companies – Mellanox Technologies is a good example, and so are Cumulus Networks and Run.ai – when it wants to do something for customers faster than it could if it had to create a technology from scratch.
About thirteen years ago I purchased three Nvidia graphics cards. A graduate student and myself went from zero knowledge to a well-performing numerical code that ran entirely on the GPU using CUDA within a semester. The program subsequently ran on a Top500 ranked cluster of K20 accelerators. After graduation the same student used GPUs to scale up a different computational problem more then 100 fold with machine-learning-based preconditioning followed by traditional scientific computation.
None of this would have happened except for the quality of the CUDA documentation, the libraries and the fact that learning could start with easily affordable consumer GPUs and no additional software expense. I expect other scientists had similar experiences since that’s the only way Nvidia could have reached where they are now.
The way I see it scalable hardware from entry level to supercomputer came together with widely available and well-documented software. Interestingly, this is exactly what led to IBM’s success with the System/360 series. I don’t think comparison with Oracle or Apple are similar.
Could not have said it better myself. Thanks.
I think Lip-Bu Tan has the best job in the world. Steve Jobs rejoined Apple when Apple was on the brink of bankruptcy. Lip-Bu Tan is rejoining Intel when Intel is in a tough spot. I hope Lip-Bu Tan can do what Steve Jobs did.
What impresses me most about NVIDIA is that they are charging ahead fast even when they are far ahead of everyone else. Compare that to Intel, which stuck with PCIe Gen 3 for 9 years: Ivy Bridge in April 2012 to Ice Lake in April 2021.
NVLink-C2C has 7x the bandwidth in each direction of PCIe x16 Gen 5 (450 GB/s vs 63 GB/s) and 1.6x the bandwidth in each direction of Intel’s latest UPI (450 GB/s vs 288 GB/s). NVIDIA is saying in the second half of 2026, NVLink-C2C will be 900 GB/s in each direction in the Vera CPU.
NVIDIA’s past R&D spending sure has paid off spectacularly. I would guess a lot of the past success of the semiconductor, computer and pharmaceutical industries has resulted from government and academic R&D, such as DARPA funding that helped create the internet.
Timothy Prickett Morgan wrote “GenAI and its foundation models are just the second wave in what will very likely be many waves of AI innovation.” I think he is 100% right. There are nearly an infinite number of ways to reorganize what is being computed with petaflops of compute. I suspect the current chips already have the performance needed for human-level AGI but we just don’t have the software yet.
There is a huge fleet of Waymo driverless taxis driving around Phoenix, San Francisco, Los Angeles and Austin. These are amazing to watch. The 6th-generation Waymo car has 13 cameras, 6 radar, 4 lidar and “an array of external audio receivers”. As impressive as this and walking robots are, I think human-level AGI will be far more impactful.
Nvidia looks like a Sun Microsystems; if you understand what I mean.
We live in a time when change happens extremely fast. Novell was a fast-rising star until came Microsoft and gave away what Novell charged a hefty price for (TCP/IP, Directory services..).
History tells me that standards always win in the end.
Mehdi Zoghlami wrote “Nvidia looks like a Sun Microsystems; if you understand what I mean. … History tells me that standards always win in the end.”
I don’t understand what you mean. Please explain. Nvidia GPUs and Cuda X are the de facto standards for accelerated computing. Most software developers don’t want to make multiple implementations of their software. Sun Microsystems got beat by x86 CPUs and Nvidia GPUs. The only thing I can imagine that could displace Nvidia would be if something had a much better price/performance ratio and a simpler programming model.
I would not accept CUDA as a standard. Maybe a de facto standard as it’s overseen by a standard body. For instance, X86 is not a standard, and so is not ARM. If CUDA was a standard, AMD would easily implement, then Nvidia would lose its luster. The same goes for NVswitch.
What defeated Sun was not a specific company or technology but its highly integrated systems and vendor lock-in. They had their moment, then the market had reconfigure itself according to mostly standard technologies. Monopolies are not sustainable in the long run.
Nvidia platform looks a lot more like Apple’s iphone than Sun’s. Both Apple and Nvidia are solving customer problems which cement their platforms broadly. Sun/McNealy took their eye off that ball when the world was changing around them. In 2025 Nvidia is driving the change in a way that customers are lined up for 12+ months to get their latest offerings.
It looks a lot more like an IBM System/360, an IBM AS/400, or a DEC VAX. Maybe an HP 3000.
Customers don’t like monopolies or duopolies. Unfortunately, the tech world is full of monopolies and duopolies that have lasted a long time. Some examples are: ASML (EUV lithography), TSMC (leading-edge foundry), Intel/AMD (x86 processors and FPGAs), Nvidia/AMD (graphics cards), Windows/macOS (consumer operating systems), Android/iPhone (cell phones), Google/Bing (internet search), Chrome/Chrome-derivatives/Safari (web browsers), Facebook (consumer social networking), YouTube (online video sharing). Big Tech are the robber barons of our time. Cory Doctorow has written extensively about the many problems caused by this situation for online products and services. See
https://en.wikipedia.org/wiki/Enshittification
I’ve monitored channel supply, trade-in and resale of Nvidia secondary ‘used’ _GPU since 2018 and Nvidia card subsystems have at least 5 lives, maybe more, handed down and resold into IT whatever (back) Nvidia standard platform generation, into world and other price sensitive commercial, academic and learning environments. There’s a lot of Xeon Skylake and Cascade Lakes resold with Nvidia back generation currently. For learning, education in economically depressed geographies people can lift themselves up learning CUDA on Nvidia hand-me-down its absolutely amazing. Here’s the currently supply, trade-in and resale trend and notice Nvidia back generation professional.
GPU Today on March 19, 2025 quick take all-inclusive percent of generation supply with sales and trade-in trend over the last 60 days and/or from the introduction of RDNA 4 and Blackwell.
KEY = Notice Nvidia Pro secondary clearing trend in relation to the RTX trade-in trend.
Nvidia Pro has 5 lives on commercial standard, CUDA student and world market utility value.
There is secondary evaluation available for AMD Pro and Instinct.
AMD = 9.39% all up card/blade share
All AMD product available in period gains + 7.8%
Mi8 to Mi210 = 0.08% + 18% that is Mi100/125/210 heavy
RDNA 4 = 0.54% + 484% in the last three weeks and average weekly gain in channel available = 156%
RDNA 3 Pro = 0.08% + 10.6%
RDNA 3 = 0.76% + 9.3%
RDNA 2 Server/Pro = 0.54% + 13.7%
RDNA 2 = 2.77% + 1.4%
RDNA 1 = 1.01% + 6.4%
Vega Server/Pro = 0.06% + 1.1%
Vega = 0.45% + 1.3%
Polaris Server = 0.21% + 4.5%
Polaris = 3.37% and flat
Intel = 0.49% all up share
All Intel in period gains + 30.6% lead by BM
BM = 0.245% + 111%
Accelerator = 0.0028% and flat
Pro = 0.0019% < 82% but in minuscule volume
Arc = 0.244% < 2.2%
Nvidia = 90.1% share
All Nvidia in period gains + 34.1%
Regardless of generation Pro is highly demanded.
On total volume Nvidia mass dwarfs AMD and Intel on trade-in.
GB RTX = 2.89% + 63% and overall + 439% from week 2 and on average + 69% per week
H accelerator = 0.15% + 25.7% shows trade-in trend
AD accelerator = 0.40% + 31.3%
AD Pro = 0.07% < 89.8% shows sales trend
AD RTX = 17.2% + 110%
GA accelerator = 0.14% < 26.8%
GA CMP = 0.11% and flat
GA Pro = 5.3% < 12.2%
GA RTX = 28.5% + 73%
TU Pro = 7.05% < 21.3%
TU RTX = 6.86% + 54.3%
TU Gf = 5.61% + 49.7%
P100 = 0.067% < 11.5%
P Pro = 5.71% < 25.9%
P Gf = 5.68% + 2.4%
MW Gf = 2.05% < 1.5%
V = 0.067% < 11.5%
Tesla mixed = 2.14% < 3%
In the last 2 months all channel available primary and secondary has increased + 31.1%
All Intel = 0.491% available in the channel today
AMD Instinct = 0.08%
RDNA 4 = 0.54%
RDNA 3 = 0.84%
RDNA 2 = 2.83%
RDNA 1 = 1.01%
Vega = 0.51%
Polaris = 3.58%
Nvidia Blackwell= 2.89%
Hopper = 0.15%
Ada = 17.67%
Ampere = 34.05%
Turing = 19.52%
Pascal = 11.45%
Maxwell = 2.05%
Volta = 0.67%
All Tesla = 2.14%
Share all up
AMD share = 9.39%
Intel share = 0.491%
Nvidia share = 90.1%
Specific mass market Nvidia Blackwell upgrade wave rolled AMD and the question is TU/GA conversion to RDNA 4?
Specific AMD how much so in HPC and Ai/ML?
Lacking conversion AMD serves its own installed base where Instinct is sales bundled with Epyc.
For mass market did AMD swamp at RDNA 4 launch? No.
Did AMD lose the rig? Yes.
The verdict is not in yet and AMD was rolled on the mass of Nvidia trade-in.
For into future commercial and HPC sales?
Turing and Ampere run end volume as a gratuity foretold the future.
Only Nvidia full runs, even in overage, sell through. Nvidia cut Ada short in my opinion to avoid the surplus dumping and foundry free license gratuity questions. This allowed a reset. I'm reflecting if that noble or conservative risk avoidance was a good business decision currently on the transitory supply void left between Ada run end and Blackwell risk into ramp volume.
Nvidia consortium Blackwell 1st degree price decision did allow AMD to enter sales channel with RDNA 4 assisted by card design manufactures raising their Nvidia price umbrellas, to let AMD into mid shelf, is the reset opportunity. Now it's up to AMD.
Mike Bruzzone, Camp Marketing