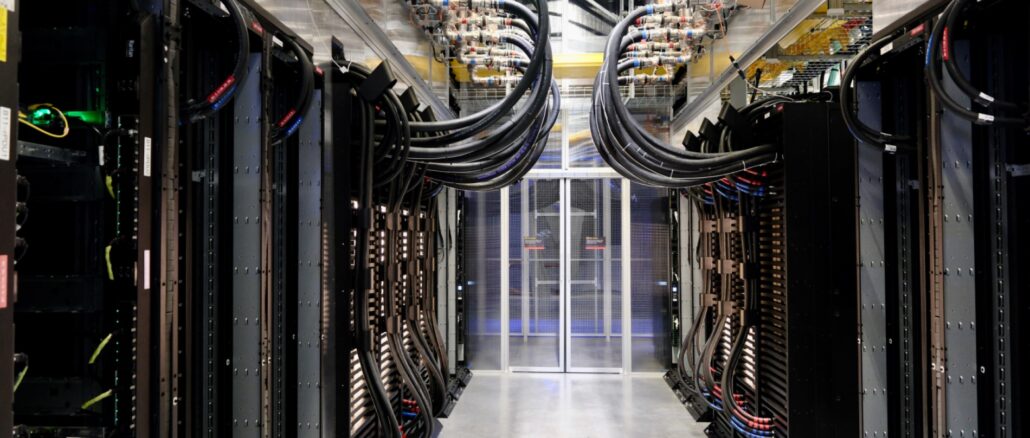
When it comes to artificial intelligence, context is everything. The same thing holds true for human intelligence, so it stands to reason that it translates to AI since we created it in our own image.
And now, we are watching companies like NVIDIA promote AI factories – literally supercomputers that think through petabytes of data and deliver intelligent answers at our prompting – as a new way to drive massive change in Earth’s global economy and cultures.
How did we get here? One step at a time, as always.
Let’s load some context before we talk more specifically about AI factories and what they mean for the future of business and society.
The Neolithic Revolution
About 12,000 years ago or so, some of our ancestors grew tired of wandering around looking for food and shelter and settled down in one place – actively growing plants and raising animals to eat. Agriculture – what we colloquially call farming – is just a food factory, and it is largely outdoors because plants and animals need sun, water, and air to grow. In medieval times, the firma was the fixed payment in rent for the land to produce crops, and hence the synonym for agriculture.
Agriculture forced us to organize people into hierarchies for farming work. Writing was created for administration – keeping track of inputs and outputs for these food factories, and ultimately the rules governing the societies that grew up around agriculture. (There were many regional varieties.) People eventually expanded the use of writing to other areas, and to this day it is still the fastest way to convey a lot of dense information.
From the moment we put down the bows and the spears and made the first hoes and rakes and plows and wrote down the first symbolic glyph in clay or carved it in stone, AI was absolutely inevitable – and so was an AI factory. It was just a matter of time. . . .
The Industrial Revolution
It took many thousands of years for humanity to get good enough at growing food surpluses that we could have the beginnings of a merchant class – people who make things for other people by hand, or manufacturing, literally “a work by hand” in Latin. This led almost instantly to a common intermediate form of exchange, or money, that accelerated bartering – the process of exchange – and transformed it into what we now know as an economy, or more precisely, the economy, since individual regional and national economies ceased to exist independently once the first wave of globalization stitched the continents back together after the age of exploration.
There have been many waves of globalization since then, which transformed both agriculture and manufacturing. The big change in factories – the place of standardized manufacturing – was the breaking down of the manufacturing process into steps to speed up production and make it more repeatable. This Industrial Revolution coincided with the Age of Enlightenment, when literacy rates soared as it became apparent that factories needed educated workers to maximize efficiency and minimize waste. Education was not a goal as much as it was a necessity, and with that education came the rights of enfranchisement and private property as well as freedom of religion, safety, and speech and the right to speedy trials by impartial juries.
We indeed hold these truths to be self-evident in the 21st century, and we have the 18th century to thank for them.
Factories took manufacturing inside. Using steam and then electricity for power and implementing techniques such as the assembly line (with people specializing in different parts of the manufacturing cycle) and lean manufacturing (cutting waste by getting parts just in time as they were needed for any manufacturing flow), we learned to make the goods of modern life so cheaply that everyone could not only afford the basics of life, but some of its comforts as well. Manufacturing took people off the farms and raised their standard of living, creating a middle class that made the economy expand in ways that an agricultural society could not have imagined.
The AI Revolution
And then the Internet happened, linking us all together and creating a new resource – data – that could be mined for insight.
The AI revolution could not have happened until a huge amount of data in the form of text, image, video and audio was computerized and until a massive amount of compute was available at an affordable price to chew on it. As we say at The Next Platform, big data is precisely enough information run against a collection of massively parallel GPUs with high memory bandwidth that is precisely enough compute to create neural networks that encode our knowledge of this world such that artificial intelligence actually works.
Let’s emphasize this: Big data is enough bits such that AI algorithms running on GPU engines can create neural networks that work.
All of these things have to come together at the same time. In the 1980s, researchers had the algorithms for neural networks, but they did not have enough compute to run them and they didn’t have enough data to feed them. So AI as we now know it remained largely theoretical until all three conditions were met.
The AI Factory: Not A Metaphor, But A Metamorphosis
The term AI factory is not a metaphor, but a literal description of what a modern AI supercomputer in a commercial setting really is. And it changes the very nature of not only corporate computing, but of data analysis – the synthesis of data and its distillation into information to compel action or inaction – as we know it.
The AI factory is inevitable, just as the agriculture factory – people working together to feed each other – was inevitable. Society and culture changed around this revolution and gave humanity free time to think and build. Now we have machines that take the sum total of human knowledge and make it not only searchable in a conversational way, but we can run the AI algorithms backwards so they can be prompted to create new data in any format.
And now, every business and every person will have an AI factory – or a time-shared slice of one – available to them. And these AI factories will create new ideas and new visions and also help us extend our individual creative capabilities.
It remains to be seen what will not be changed by the advent of these AI factories. We can’t think of anything that won’t be. And neither can the chatbots if you ask them, or the makers of the parallel compute engines that train the models and execute the inference that drives the AI models, or the model makers themselves like OpenAI, Anthropic, Google, Mistral, and many others. There may not be a lot of consensus on Earth about many things these days, but anybody who is paying attention to the AI revolution agrees that everything we know and do will be affected by the increasing sophistication and relevance of AI.
Where Insight And Action Is Manufactured
The AI factory has two jobs. The first is to train the foundation models that provide the insight we all seek to improve our businesses and our lives. The second, and ultimately more important, job is to feed new data and questions into a model and have it infer new answers – generate new tokens – to provide further insight and to drive action.
For the past decade, a lot of the discussion around AI has been around the training of ever-embiggening foundation models, now with hundreds of billions to more than a trillion parameters (akin to synapse spiking levels in the human brain) and the amount of data (trillions to tens of trillions of tokens, and growing). As we have pointed out before, token counts tell you how much you know, and parameters tell you how well you can think about what you know. Smaller parameter counts against a larger set of tokens gives you quicker, but simpler, answers. Larger parameter counts against a smaller set of tokens gives you much better answers about a limited number of things. And these days, chain of thought reasoning models, which are also multimodal in nature and not just focused on text, are stitching together many hundreds of specialized models so they can work together, considering outputs that drive other inputs, to take more time and generate better token streams we humans call answers.
With the AI factory, all of the content created by humanity and synthetic data generated by AI models is the raw material. Insight derived from that massive historical trove of data is what is harvested and people with human intelligence and agents with artificial intelligence make use of that insight to do stuff. This time, people don’t go to the factory to work, but they tap into that factory as part of their work, augmenting the wide knowledge and speed of the models with their own skills to do more things better and faster.
“The world is racing to build state of the art, large scale AI factories,” Jensen Huang, co-founder and chief executive officer at NVIDIA, explained in his keynote address at the recent 2025 NVIDIA GTC in San Jose. “Bringing up an AI factory is an extraordinary feat of engineering, requiring tens of thousands of workers from suppliers, architects, contractors, and engineers to build, ship, and assemble nearly 5 billion components and over 200,000 miles of fiber – nearly the distance from the Earth to the Moon.”
Building an AI factory is a significant capital investment, as you might imagine. A reasonable configuration of an AI factory is an NVIDIA DGX SuperPOD based on eight racks of DGX B200 systems, which is comprised of GPUs, CPUs, Quantum-X InfiniBand or Spectrum-X Ethernet interconnects between the nodes, and storage.
With 32 of the DGX B200 systems, this SuperPOD delivers 4.61 exaflops of FP4 performance against 48 TB of HBM3 memory with 2 PB/sec of aggregate memory bandwidth. As you need more performance, you can scale out from there.
Another blueprint for an AI factory from NVIDIA is based on the NVIDIA GB200 NVL72 platform, which is a rackscale system that also includes GPUs, CPUs, DPUs, SuperNICs, NVLink and NVSwitch, and InfiniBand and Spectrum-X networking, but which presents a much larger shared GPU memory domain for AI models (72 GPU sockets compared to eight for the DGX B200 nodes) and which has much higher compute density and therefore requires liquid cooling.
The GB200 NVL72 was launched in March 2024 and is shipping in full volume now. That rackscale system is indeed a system – it doesn’t need anything but your data to get to work building a model and then turn around and start kicking out tokens of data in text, image, video, or sound formats.
The basic building block of the GB200 NVL72 is an MGX server node that has one NVIDIA Grace CPU working as a host processor for a pair of Blackwell GPUs, which are themselves a pair of Blackwell GPU die in a single SXM socket. Two of these server nodes are combined into a compute tray built into the NVL72 rack. There are 18 compute trays in the rack that make up 72 GPUs (144 GPU die) and 36 CPUs.
The GB200 NVL72 rackscale system combines Grace CPUs attached to a pair of Blackwell GPUs, with 450 GB/sec NVLink connections between the CPU and the GPUs. The 1.8 TB/sec NVLink ports are used along with NVSwitch chips to link all the 72 GPUs together (144 GPU die with 900 GB/sec each) in an all-to-all, shared memory configuration that is perfect for foundation model training (when they are interlinked for massive scale) and also for chain-of-thought inference.
The NVLink fabric, created by nine NVLink switch trays with a total of eighteen NVSwitch chips, allows for those 144 GPU dies to be accessed like one giant GPU as far as AI applications are concerned.
The GB200 NVL72 systems sport 2,592 Arm cores for host processing, and 1.44 exaflops of floating point processing at FP4 precision, with the throughput cut in half each time you raise the precision by 2X. The GB200 NVL72 system has 13.4 TB of HBM3e memory attached to the GPUs, with up to 576 TB/sec of aggregate bandwidth. Those Grace CPUs have a total of 17.3 TB of LPDDR5X memory that is just one NVLink hop away and that has another 18 TB/sec of aggregate bandwidth.
The NVIDIA GB200 NVL72 is to the AI revolution what the System/360 was to the online transaction processing and batch processing revolution five decades ago. One big difference between then and now is that the NVL72 can be scaled out through InfiniBand interconnects, which is exactly what happens in a DGX SuperPOD, but once you bought the biggest System/360, that was it. You had to wait for the next upgrade cycle to get a more powerful machine.
The DGX SuperPOD configuration based on the NVL72 rackscale systems requires nearly 1 megawatt of power, but delivers 11.5 exaflops of computing and 240 TB of HBM3e memory across eight compute racks. If you need more performance, as with any SuperPOD, you just add more racks.
The compute density of the NVL72 rack requires specialized liquid cooling and datacenter features to support that liquid cooling. By the way, liquid cooling is not a new idea in terms of either technology or economics, and in a way, the use of liquid cooling is a return to the past when big iron machines that transformed every business in the 1960s and 1970s were also water-cooled so they could drive the absolute maximum performance available at the time. So in a sense, liquid cooling demonstrates how serious we are about maximizing performance and efficiency out of datacenter infrastructure.
An AI factory will almost certainly need much more computing than this as inference gets embedded in all manner of applications, particularly if you want reasonable query and agentic AI performance and as we inevitably shift to chain of thought reasoning models, that are estimated to take 100X more compute than simple one-shot, blurty answers common with earlier large language models.
An AI factory is not just hardware, but a slew of systems and development software and services that make the hardware useful.
DGX GB200 systems and related DGX SuperPOD AI supercomputers need to be managed and modeled, and that is where a few different tools come in. NVIDIA Mission Control, including Run.ai, orchestrates AI workloads across the infrastructure and also recovers jobs automatically when issues arise. Mission Control does health checks on the system and helps optimize power consumption against the workloads running on the systems.
On top of this is NVIDIA AI Enterprise, the systems software that includes libraries, models, and frameworks that have been optimized for acceleration on NVIDIA GPUs and networks. That AI factory stack now also includes NVIDIA Dynamo, an open source, distributed framework for running inference across NVLink and DGX SuperPOD infrastructure. DGX Expert Service and Support helps customers implement these technologies quickly and reduce the time to first token coming out of their AI factories. And for those who build and expand these systems, NVIDIA has created AI factory blueprints for its Omniverse “digital twin” environment and design tools to simulate the entire datacenter that comprises an AI factory so it can be built right the first time and be kept right as it is inevitably expanded.
Perhaps the most important aspect of an AI factory is the shift in thinking that it engenders, and the focus that NVIDIA is making in its current systems and those on its roadmap, which gives customers assurances that there will be plenty of headroom to grow rackscale and pod systems.
“I think what is driving a lot of the excitement and demand for AI factories is that generating tokens now equates to generating revenue for a lot of companies,” says Gilad Shainer, senior vice president of networking at NVIDIA. “We are not looking at a datacenter as a cost center, but as a productive asset that is going to generate revenue.”
And that, after all, is what building a factory is all about.
This content was sponsored by NVIDIA.
You make me think of Vonnegut’s “Player Piano”
The modern factory was conceived by Ford. Womack, Jones and Roos (1990) commented “To achieve interchangeability Ford insisted that the same gauging system be used for every part all the way through the entire manufacturing process.. Remarkably.. no-one else pursued working-to-gauge with Ford’s near religious zeal.” My point, actually progress throughout human history is built on precision, repeatability, interchangeability. Every AI model is imprecise, it’s outputs are not repeatable and as each model creates its own opaque black box parameterisation of the world no two models have any interchangeable parts. The current generation of AI is a massive step backwards and demonstrates a lack of understanding of history.