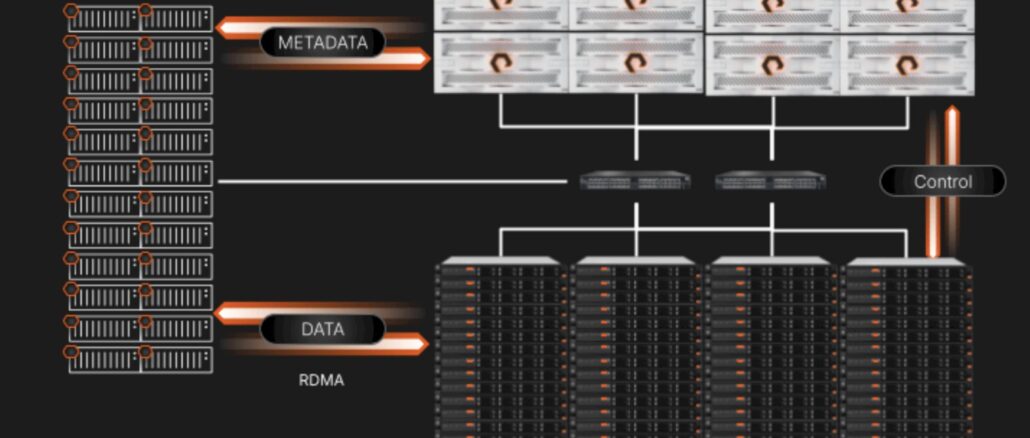
The injection of generative AI into the bloodstream of the tech titans and now businesses of all sizes and stripes over the past two years has forced IT vendors, from hardware makers to component providers to enterprise application developers, to quickly rework their roadmaps to address the particular demands of and opportunities presented by this emerging technology.
This includes a bevy of data storage system makers, who had already addressed scalability challenges brought on by HPC centers and other organizations operating compute at scale. But those storage systems – including parallel file systems and disaggregated storage – didn’t necessarily translate to the needs of AI environments, according to Chadd Kenney, vice president of technology at Pure Storage.
With AI, workloads are less predictable than more traditional applications, they are complex and they are multimodal – chewing on and outputting text, images, and video – Kenney tells The Next Platform. The performance and scalability were there for those existing workloads, but they didn’t work well for AI environments that include thousands to tens of thousands of expensive GPUs crunching through these applications. Organizations were telling Pure Storage they weren’t fully able to utilize all the GPUs these had and that performance – essentially the ability to read and write in a consistent manner – and scalability were suffering because of what they saw as storage bottlenecks.
“Many of them started to think about the fact that, as these models are developing and they are becoming more multimodal, it is no longer just text-based,” he says. “We’ve got all these different types of media now that these systems have to process, which is very different than the traditional HPC workload. While there were differences in those types of workloads, they tend to be relatively consistent in the way that the data was being processed.”
There also was the growing complexity, from the need for multiple different namespaces or new types of products for different parts of the pipeline, all of which were contributing to high costs and low ROI.
For Pure Storage, most of the pain was being felt in a particular market segment. Its scale-out FlashBlade storage platform was being used by hundreds of organizations for enterprise workloads, Kenney says. Likewise, Pure Storage recently announced a design win with Meta Platforms, its first with a hyperscaler and giving the company a foothold in the ultra-high-end, where organizations run tens of thousands of GPUs in their systems and need to keep them fed.
But it was customers in the middle part of the market – those running between one and tens of thousands of GPUs and who wanted performance of 1 TB/sec to 50 TB/sec out of their storage arrays, and who are building what Nvidia calls AI factgories – where Pure Storage and pretty much everyone else hadn’t done much, according to Kenney.
“The people who are buying most of the GPUs today are training at massive scale,” Kenney explains. “We wanted to know about the requirements of these customers. We knew what the enterprise requirements were. A terabyte per second would get you most customers out there because they were still in early experimental phases. There were typically less than a thousand GPUs for most of the enterprises that we had seen out there. Hyperscalers were on the ultra-high end. They wanted 50 terabytes per second-plus, and they were building completely different architectures. We learned so much from them during the hyperscale design win that made us start to think about our products a little bit differently than how we had before.”
That thinking led to the introduction this week of Pure’s FlashBlade//EXA, a storage platform built atop the FlashBlade infrastructure with a focus on high concurrency and the huge amounts of metadata that are found in HPC and AI workloads and that have been the cause of a lot of storage bottlenecks that choke AI performance. FlashBlade//EXA addresses that by scaling data and metadata independently.
There were two concepts Pure Storage looked at when trying address the metadata challenge. One was the parallel file systems, built for HPC and good for scalability and performance, but complex in terms of upgrading a thick client and managing the system. The other was disaggregation, which also pre-dated the current AI era and came with its own performance bottlenecks, particularly around writes, with the metadata and data ingest being done on the same tier.
Pure Storage engineers knew metadata was highly optimized in FlashBlade, but the vendor wanted to allow for endless scale for data nodes, ease of adoption with traditional protocols, and networks to let enterprises bring it easily into their environments, Kenney said.
“We built this FlashBlade//EXA architecture using FlashBlade as the metadata core and then utilizing data nodes for ultra scalability and allow you to flexibly increase in any direction that you want,” he says. “You can have ten data nodes and a whole bunch of metadata if you really need it, if you have tiny, tiny files. You could have many data nodes and only one metadata node. There’s no constraints on the scale model of this. The platform now becomes this extension of FlashBlade.”
The highly disaggregated and parallel architecture will eliminate the performance impact of multimodal models and will start with 10 TB/sec read performance in a single namespace he added. In addition, organizations will be able to use some off-the-shelf technology – it’s compatible with data nodes from other storage makers while FlashBlade will be used for the metadata – along with Pure Storage’s own technologies.
“We’re going have these data nodes being off the shelf because customers asked for this from the very beginning when we started talking to them about potentially pulling this off,” Kenney said. “They wanted to leverage existing investments right away.”
The plan is for Pure to offer a data node built on its DirectFlash Module (DFM), starting at 75 TB and 150 TB and later 300 TB, which will deliver between 1.8 PB and 7.2 PB in a 2U model.
Pure this month is starting to let customers start testing out the platform, with about a dozen or so running proofs-of-concept, and plans to make it generally available in the summer. Initially the system will support pNFS for access, with plans to provide S3 over RDMA coming soon after, along with DFM data nodes, Nvidia certifications, and integration with Pure’s Fusion storage-as-code services.
Be the first to comment